Artificial intelligence (AI) has the potential to revolutionize the agricultural industry in the coming years. With the world’s population projected to reach nearly 10 billion by 2050, the demand for food is only going to increase. AI can help farmers produce more food efficiently, sustainably and with less waste, while also improving the quality of the food produced. In this article, we will explore how AI is changing the future of agriculture.
Precision Agriculture
Precision agriculture is a farming technique that uses technology to monitor and manage crop growth. It involves collecting data about the environment, such as soil moisture levels and weather conditions, and using this information to make precise decisions about how to grow crops. With AI, farmers can collect more data and make more informed decisions about when to plant, water, fertilize and harvest their crops. By doing so, they can optimize crop yields and reduce waste.
One example of precision agriculture is using drones to monitor crop growth. Drones can fly over fields and take high-resolution images of crops, which can be analyzed using AI to detect plant stress, pest damage or nutrient deficiencies. Farmers can then use this information to make targeted interventions, such as applying pesticides or fertilizers to the areas that need them most.
Another example is using sensors to monitor soil moisture levels. Sensors can be placed in the soil to measure moisture levels at different depths, which can help farmers make decisions about when to water their crops. AI can analyze this data and provide recommendations about how much water to use and when to irrigate. This can help farmers save water and reduce the risk of over- or under-watering their crops.
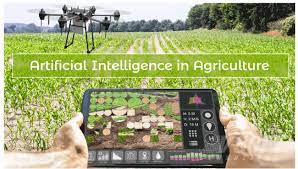
Plant Breeding
Plant breeding is the process of selecting and breeding plants to create new varieties with desirable traits, such as higher yields, disease resistance, or improved nutritional content. Traditionally, plant breeding has been a slow and laborious process, taking years or even decades to develop new varieties. With AI, however, plant breeding can be accelerated, allowing for the creation of new varieties in a fraction of the time.
One way that AI is being used in plant breeding is through machine learning algorithms that can analyze large datasets of genetic information. By analyzing the genetic information of plants, AI can identify genes that are associated with desirable traits and use this information to create new plant varieties. This approach is known as genomic selection, and it has the potential to revolutionize plant breeding by accelerating the development of new crop varieties.
Another way that AI is being used in plant breeding is through CRISPR/Cas9 gene editing. This technique allows scientists to precisely edit the genetic code of plants, creating new varieties with desirable traits. AI can be used to predict the effects of different genetic modifications, allowing scientists to make more informed decisions about which modifications to make.
Smart Irrigation
Smart irrigation systems use technology to monitor and control the amount of water used to irrigate crops. By using sensors and AI, smart irrigation systems can adjust water usage based on real-time data about soil moisture, weather conditions, and crop water needs. This can help farmers save water and reduce the risk of over- or under-watering their crops.
One example of a smart irrigation system is using weather data to predict when and how much water to apply to crops. By analyzing weather patterns, AI can predict how much water will be lost to evaporation and adjust irrigation accordingly. This can help farmers save water and reduce the risk of waterlogging or drought stress.
Another example is using sensors to monitor soil moisture levels and adjust irrigation accordingly. Sensors can be placed in the soil to measure moisture levels at different depths, which can help farmers make decisions about when to water their crops. AI can analyze this data and provide recommendations about how much water to use and when to irrigate. This can help farmers save water and reduce the risk of over- or under-watering their crops, which can lead to reduced yields and poor crop quality.
Crop Monitoring and Management
AI can also be used to monitor and manage crops, helping farmers to detect problems early and take corrective action. For example, AI can be used to detect pests and diseases in crops, allowing farmers to take action before they spread and cause significant damage.
One example of using AI for crop monitoring is using computer vision algorithms to analyze images of crops. By analyzing these images, AI can detect patterns and anomalies that may be indicative of pest or disease infestations. This can help farmers detect problems early and take action before they cause significant damage to crops.
Another example is using AI to monitor crop growth and predict yields. By analyzing data about soil moisture, weather conditions, and other factors, AI can predict how crops will grow and how much they will yield. This can help farmers optimize their management practices and ensure that they are getting the most out of their crops.

Food Safety and Quality
AI can also be used to improve the safety and quality of the food produced by the agricultural industry. For example, AI can be used to detect foodborne pathogens, such as salmonella and E. coli, in food processing facilities. By using machine learning algorithms to analyze data about food processing and storage conditions, AI can identify potential sources of contamination and help prevent outbreaks of foodborne illness.
Another example is using AI to improve the nutritional content of crops. By analyzing data about soil conditions, weather patterns, and other factors, AI can identify the optimal conditions for growing crops with high nutritional content. This can help farmers produce healthier crops and improve the nutritional value of the food they produce.
Challenges and Concerns
Despite the potential benefits of AI in agriculture, there are also challenges and limitations to its implementation. One challenge is the cost of implementing AI technologies. Many AI technologies require significant upfront investment, which may be a barrier for small farmers or those in developing countries.
Another challenge is the availability of data. To be effective, AI requires access to large amounts of high-quality data. In some parts of the world, data on weather, soil, and crop yields may be limited or unavailable, making it difficult to implement AI technologies.
Finally, there are concerns about the ethical implications of AI in agriculture. For example, some have raised concerns about the use of gene editing technologies like CRISPR/Cas9, which could have unintended consequences for biodiversity and ecological systems. Others have raised concerns about the potential for AI to exacerbate inequalities in the agriculture sector, with large corporate farms benefiting at the expense of small farmers.
Conclusion
AI has the potential to revolutionize the agriculture industry by improving efficiency, sustainability, and reducing waste. Precision agriculture, plant breeding, smart irrigation, and crop monitoring and management are just a few examples of how AI can be used to optimize crop yields and improve the quality of the food we produce. However, challenges and limitations must be addressed, including cost, data availability, and ethical considerations. With careful implementation and thoughtful consideration of these challenges, AI has the potential to create a more sustainable and efficient agriculture industry that can meet the growing demands of a rapidly expanding global population.